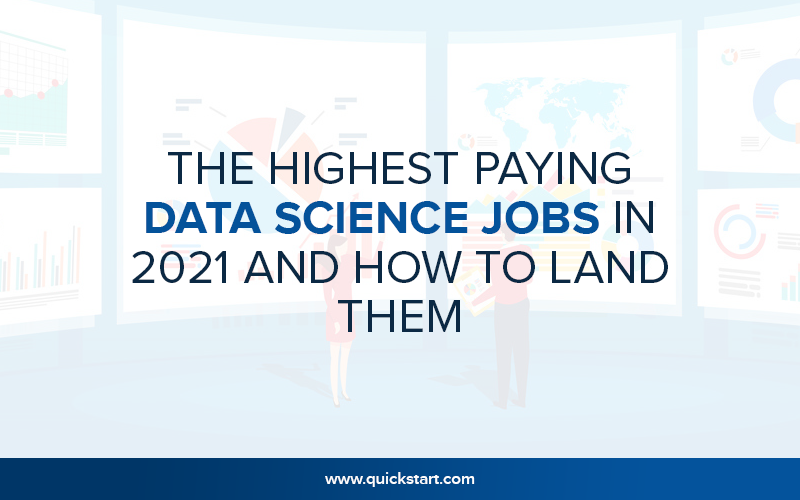
The Highest Paying Data Science Jobs in 2021 and How to Get One
These days, data science requires the expertise of professionals who can collect, organize, store, process, and analyze data, enabling individuals and organizations to make decisions based on data generated from data. All the same, data experts are among the highest-paid resources in the IT sector. Data science is fast becoming a market because companies are always looking for simpler ways to access the huge amount generated by the Internet of Things (I-o-T) devices.
Combining ingrained statistics and mathematics with the modern disciplines of data analysis, and Machine Learning - data science can provide the key to companies. However, a direct challenge for companies is that the demand for data experts is greater than the supply. Re-application and skills development programs can help knowledge-based companies build their fund of talented professionals.
Who Is a Data Scientist?
It is believed those data scientists are individuals with extensive knowledge of data analysis, sophisticated problem-solving skills, and the curiosity to investigate many new cases. They are considered the best in both industries - information technology and business - and are therefore highly qualified individuals with a professional role in the world of information technology, statistics, and analytics development. Due to the growing demand for personal analysis and exploration in various technical disciplines like artificial intelligence and data science, data scientists enjoy higher than usual salaries.
The field of data science has grown greatly in a very short time as companies understand the importance of collecting large amounts of data from websites, devices, social networks, and other sources and using them for business purposes. According to the study, the number of data scientists and computer experts is expected to increase by 15% by 2028.
Topmost Data Science Job Roles
Data science professionals are in high demand in almost all industries and are not limited to the IT industry.
Data Scientist
It is considered that this is the most demanding role these days to work with data. They help identify machine learning trends, predict data development, and write code to facilitate data analysis. Data analysis typically works in conjunction with companies or management team teams to identify a company’s needs.
Data Analytics Professional
An IT professional should have skills such as Data Viewer, Microsoft Excel, R, SQL, etc. With little experience, training, data analytics certification, and knowledge, candidates who want to become a data expert can embark on a world of analysis. Performs machine tests and experiments and applies appropriate ML algorithms.
Business Analyst
Business professionals differ from other professions. Business professionals act as a bridge between data processing engineers and managers. They should understand corporate finance, business information, as well as computer technology such as data models, data analysis tools, and so on.
Data and Analytics Leader
The data and analysis leader coordinates the various projects of his team for large data projects. Communicate with heads of different business departments to develop policies and coordinate goals. They are also supposed to work closely with the team of front and rear mechanics, product managers, and experts, and defines the company’s data assets, sparks, spark SQL, and hive SQL to run data models.
Data Analyst
They are supposed to get training from the data analytics Bootcamp and collect, evaluate and interpret data to help make many business decisions. They identify data development and links, design data collection processes, and distribute results to stakeholders. With expertise in math and statistics, they also need strong communication skills to communicate the results of their analysis to others in their organization.
Key Reasons to Become a Data Scientist
Becoming a data scientist is the dream of many data enthusiasts. However, here are the core reasons to be a data expert:
High Demand Industry Area
Data exploration is considered one of the most popular jobs by 2020, and one estimate is that it is expected to create about 11.5 million jobs in the area by 2026. Demand for scientific data is growing, while supply is too low. The lack of qualified experts with Data science training has increased market demand. All the same, a recent study shows that 45.3% of companies lack data analysis skills as a major challenge for their growth.
Highly Professional
As data science is key to the decision-making process, companies are willing to hire more data researchers who obtained training from data science Bootcamp to help them make the right business growth decisions. As this is a less saturated area with medium skills, a variety of opportunities have emerged that require a variety of skills.
Developing Workplace Environments
With the advent of technologies such as artificial intelligence and robotics, the vast majority of manual tasks have been replaced by automation. However, ML has enabled the data analytics training of machines to perform repetitive tasks and allows people to focus on important problems that need their attention. Many new and exciting technologies have emerged in this field, such as blockchain, edge computing, server without a computer, and the like.
Successful Product Standards
Detailed use of algorithms to learn regression, problems with classification proposals such as decision tree, random forest, neural network, naive Bayes increase the desired user experience for companies. One of the best examples of this development is online shopping sites that use smart referral systems to target products and provide customer-focused information based on their past purchases. Counter-investigators serve these companies as trusted advisors, defining the chosen market auditor and managing marketing strategies.
Serving the World
In today’s world, almost everything is data-driven. Data experts with data science certification work with confidential data from vast databases to help make decisions in industries, from finance and healthcare to manufacturing, pharmacology, and engineering. Organizations possess data-based knowledge that increases productivity and growth while optimizing capital and reducing potential risks.
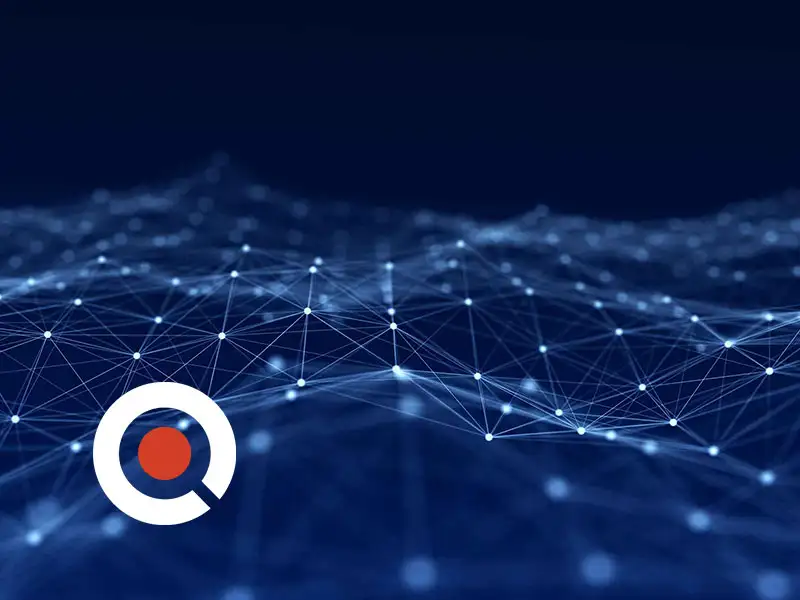
Introduction to Data Science
On DemandThis is the initial step in the Data Science educational plan from Microsoft.
Explore CourseSkills Needed by a Data Scientist
We need to be aware of the basic knowledge that consists of a data scientist:
- Understanding data - as the name suggests, data science refers to data. You need to understand the language of the data, and the most important question you need to ask yourself is whether you enjoy working with data and analyzing numbers. And if your answer is yes, you are well on your way.
- Understanding algorithms or logic - algorithms are a set of instructions that a computer receives to perform a specific task. All machine models are based on algorithms, so understanding them is an important prerequisite for a future data scientist.
- Understanding programming - you don’t have to be a specialist to become a data science specialist. You should still have basic programming information that includes variables, constants, data types, conditional statements, IOs, client or server, database, APIs, hosting, and so on, it’s good to go.
- Understanding statistics - data is one of the crucial and critical domains of the exploration. You should be familiar with concepts such as mean, median, mode of operation, standard deviation, distribution, probability, Bayesian theorem, and various statistical tests like theories, kefir, and so on.
- Understanding the field of business - if you do not have a thorough knowledge of the field of business, this is not an obstacle to your data researcher’s journey. However, if you primitively understand a particular industry in which you work, it also has additional benefits that can take you forward.
With all of the above, you need to have good communication skills to help the whole team get to the same site and work well together.
Data Science Needs New Thinking
Process automation - however, information differences still exist for many companies. When the amount of data collected explodes, the entire company processes existing systems and yields valuable results. There’s not a lot of it, but employees can’t use the data. As a result, many organizations have little choice but to focus on narrow data - an insufficient share when responses require a larger share of the total.
A growing category of automated analytical processes, or APAs, may be the key to achieving the best in man and machine. The APA automates business processes and provides new information officers with direct self-service access to critical fast data. In practice, this means that more employees can obtain data and use it without more training. This in turn breaks the familiar tension between data scientists and business leaders, who rely on them to access the information they need.
The data architecture is a platform for standardizing the collection and dissemination of data between companies. It provides users with access to high-quality data quickly and at a relatively low cost. This lays the foundation for making important day-to-day business decisions. Data architecture in enterprise architecture is data planning used to define the target situation and further planning is needed to achieve the target situation.
It is usually one of the many building blocks that make up the backbone of architecture. A real company has a very complex data subject. Most data is stored in older systems or large packages whose data structure information may be unknown. Other information is stored in spreadsheets and databases and may be invisible to the company’s information technology or core data services.
Late development - with an integrated approach to data science management, business process automation, and employee engagement with strategic solutions, APA shapes how companies create data-driven knowledge and implementation. It allows craftsmen in all areas of the company to ask difficult questions and quick answers, always relying on advanced IT skills.
These innovative technologies are helping companies create new products and services while also finding ways to target supply chains and businesses. The demand for data scientists continues to grow. Any company, organization, or entity that creates or uses data can and has already invested in data experts to help them excel in today's challenging economy.
Why Is Data Aggregation in Data Science Important?
When compiling and designing data, you take important steps to achieve the best results for your goals. In most cases, this is something like profitability or a measure of your actual financial income based on market costs and other costs. For example - request that you provide the service and that the customer come to your site. In this example, it is easy to track data science aggregation through and determine the return on investment.
This, in turn, helps us understand the value of marketing. This is especially useful if you use two similar sources and want to know which one provides the most value. However, the course through which raw form of data is supposed to be collected and expressed in a concise form for statistical analysis. For example, new data may be collected over some time, including statistics such as total, average, maximum, minimum. You analyze aggregate data after collecting and writing data for visual purposes or for reporting purposes to obtain valuable information about specific resources or groups of resources.
Although the latter option may seem easier to the user, it is necessary to calculate and compile the amount of data to be available to you this time. The importance of data science is growing as technology is increasingly integrated into our lifestyles, both at home and at work.
Based on specific demographic or behavioral characteristics aggregate data are widely used in statistical analysis to collect data on specific groups. Consumer data can be collected from a variety of sources, such as Internet browsing history, social media, and other personal information, to provide customers with the necessary information.
That means removing duplicates and variations of the same data you have, so you only have one gold copy or so few copies. However, manual data processing requires resources and shows the risk of human error. Pre-prepared data is published so that it can be used later - that is their purpose. If necessary, you must also document the actions or logic used to interfere with those actions. Fewer mistakes mean happier customers and less frustrated employees. Higher productivity and better resolution are other benefits of it. This removes major unavoidable errors and inconsistencies when combining multiple data sources into one data packet.
Inaccuracies When Processing Data by Data Scientist
Some common errors in organized data are missing in the fields. These errors can be corrected using tools such as Google’s tested structured data tool. This tool gives you a list of all the errors and detailed information about the organized data that Google now finds on your site. Deleting, duplicating, inaccurate, or inaccurate data can cause costly interference; if the event is not considered normal, it should be excluded from the analysis.
Comparisons of different population groups, sections, or clusters can also lead to inconsistencies in data by data scientists. The same goes for collecting high data. Another error that can occur by data scientists is when the wrong programs are received from libraries. Once the data cleansing process is complete, the company can safely move on and use that data to gain a deeper insight into the business.
However, there are many other reasons for carrying out this procedure, all of which are very useful. On the other side, the amazing element that common data means that databases take up less space. As technological advances in storage become more efficient, we are now entering an era in which gigabytes, terabytes, and more will no longer shrink. As such, finding ways to reduce space is paramount and the usual data can do.
Why Should Data Scientist Approach Problems in an Expected Way?
Special thinking goes hand in hand with advanced analysis. Unlike a responsive SQL query, a special thought process is required. For example, consider prediction analyzes before closing. Business Intelligence (BI) and SQL processes can generate reports on customers who have left the service. A control panel may be available showing how many people have changed service providers, how much they spent on the service per month, and so on. However, the expected approach tries to predict the probability of the customer leaving the service.
A proactive and predictive approach requires different thinking about the problem. The goal or outcome of the interest must be examined - in which case is the service terminated or maintained? By using relevant (potentially derived) user attributes such as billing information, purchase history, length of stay as a customer, demographic data, even geology, or other data such as text data, you will get a tool training set. The result contains classification rules that include, in the case of a decision tree, the probability of leaving or leaving the customer service. You can then use the test database in the algorithm to see how it works compared to the new data. If it works well, you can use a template.
The magic is to change the thought process and look for customer characteristics that behave in a certain way, not just ask a query to show customer behavior. The ability to communicate results is a key to storytelling that involves analyzing data science. The story must exceed the direct narration of facts to incorporate analytical factors that have an impact. However, an introduction or a modern story with maps can be updated as the data changes. These stories should arouse the interest of the audience and arouse emotionally. Developing communication skills is an important consideration for professionals. Build an indoor pool of talent - these plans, while ensuring the sustainability of a company’s growth, can also be a lifeline for employees.
Data Science Significance in 2021
Thanks to the growing range of data, data science has reached a stage in the interdisciplinary field. Data science is a concept that combines ideas, data observation, machine learning, and related methods to understand and attribute phenomena applied to data. A data scientist works with a huge amount of data to process important information with a huge amount of data and identify suitable models and designs so that they can be used to determine future goals. The standing of a data scientist can be determined by some of the reasons on behalf of which it is growing.
- When products and companies make full use of this information, they can share their stories with their audience and thus better connect with the products.
- Data science is now available in almost all areas, and there is a lot of data in the world today and, if used properly, it can lead to product success or failure. If the data is used properly, it is important to achieve future product goals.
- Small data is created and constantly changed. Using a variety of regularly developed tools helps a large data business to effectively and efficiently solve complex IT, human, and resource problems.
- Data science is gaining popularity in all industries and therefore plays an important role in the operation and growth of any product. Therefore, the demand for IT is increasing, which must perform important tasks in data processing and offer solutions to certain problems.
- Data science has also influenced retail. Let’s take an example to understand this; seniors had a lot of contact with a local trader. The seller was also able to respond to customer requests in a personal way. But now, with the advent and rise of the supermarket, that attention has disappeared.
Data science has affected different areas. Data Science helps organizations communicate with customers. It shows that the popularity and importance of data scientists are growing. New approaches and methods are constantly being discovered in every single industry, and people need better care. Data science produces better opportunities for learners to improve their knowledge. It offers the best solutions to meet the challenges of increased demand and a sustainable future.