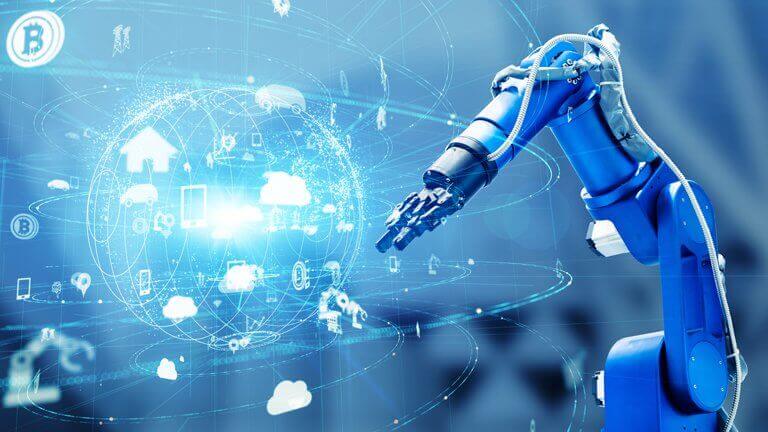
Earning a Top Machine Learning Career in 2022
As the world grows evermore digital, the demand for machine learning experts expands in parallel. In this era, technology companies, especially large ones, pay their employees based on how well they can leverage machine learning to save time, money and effort. Today, machine learning (ML) skills are among the highest prized abilities to characterize any resume. According to the International Data Corporation, spending on Artificial Intelligence and ML has already increased exponentially.
Suffice to say, the demand for skilled machine learning experts has never been higher.
What is machine learning?
As a subdomain of artificial intelligence, machine learning allows computers to learn independently, without individual programming. Machine learning can also refer to an algorithm that receives, isolates and converts data into useful functions.
This technology involves the learning of machines to perform certain tasks. Machine learning professionals create programs called algorithms (problem-solving rules) to complete tasks and predict future trends.
The best jobs in machine learning in 2022
Depending on your individual skills in machine learning and artificial intelligence, you might be better positioned to succeed in certain machine learning careers. Let's examine some of the most popular, highest-paying ML career options below.
Data engineer
Your organization's data engineer is a key member of the analytical team. Data engineers use technology to automate business processes, and work with and raw data and classification parameters. Data engineers are also responsible for statistical analysis of source data, their aggregation and the transfer of relevant information. Data engineers require coding skills to calculate the sheer volume of data they regularly filter.
Machine learning analyst
Another demanding job in ML is machine learning analysis. Aspiring ML analysts should study data assessment best practices and use modeling techniques to better assess information. However, you'll also need practical experience working with statistical models, and the ability to diagnose complex problems and follow a diagnostic method to solve them. Machine learning analysts must be specialized in data processing, and also review statistical programming languages.
Data analyst
Most organizations expect data professionals to become familiar with data recovery and storage systems, data analysis and ETL data storage tools, based on Hadoop analysis and business intelligence ideas. Data analysts clean, manage and organize large amounts of data from a variety of internal and external sources. The main tasks of a data analyst include the design and use of algorithms, data removal and risk recognition, data extrapolation through advanced computer modeling, error codes mitigation and data decisions.
AI architect
Although deep learning tends to dominate, the combination of ML and artificial intelligence is also one of the better-paid jobs in mechanical engineering. AI architects test, support and implement quality products used by different companies, helping them more informed decisions. Artificial intelligence combined with machine learning improves the candidate’s abilities, and at the same time takes advantage of the quality of work that the candidate offers.
Machine learning engineer
Many organizations want to increase the capacity of their workforce by introducing machine learning algorithms, products and solutions. To meet industry standards and improve business, companies hire machine learning professionals. Machine learning engineers work closely with research and development teams to create and implement systems that are capable of improving overall quality of service. They have the power to create unique models to solve deep data challenges, leveraging mathematical experience to assess ML performance.
Data architect
Data architects are responsible for continually optimizing a company’s comprehensive data ecosystem. A data architect also develops custom analysis software and software components. They collect and store data, perform real-time packet processing, and serve it for analysis by a computer engineer via an API. Data architects are also experts in big data technology and analysis tools. They use coding to sort large amounts of disorganized data to gain insights and help shape future policies.
Industries that use machine learning
The use of machine learning goes far beyond the technical world. For example, financial services use ML to identify investment opportunities, explain trading time to investors, identify risk-averse clients and detect fraud. In healthcare, algorithms help diagnose disease by proactively identifying abnormalities.
Machine learning is also prevalent in advertising and marketing spaces. ML enables the marketing and sales industry to identify consumers based on their procurement and research history.
Key skills required for machine learning jobs
Before you can fulfill a rewarding career in machine learning, you'll need to develop a few foundational skills. These skills help you fulfill key ML tasks on a daily basis.
Applied math and used algorithms
With a solid understanding of algorithm theories and knowledge of how algorithms work, you can also differentiate models like SVM. You'll need to understand topics such as true slope, convex optimization, displacement, exponential programming and differentials.
Basics of programming and CS
ML involves computing in large databases; therefore, strong skills in computer architecture, algorithms, data structure and complexity are required.
Statistics
Tools and graphs are very important in machine learning for creating data models. Since machine research algorithms are based on statistical models, the importance of statistics in machine learning can't be overstated. Thus, statistics play an important role in the development of algorithms. Therefore, it is necessary to acquire knowledge of statistical equipment to speed up the mechanical engineering process.
Probability
Probabilities helps predict future business performance. Mathematical probabilities, such as derived techniques and Bayesian networks, help mechanics predict the future. The official description of probabilities (conditional probability, Bayesian rule, probability, independence, etc.) and the resulting methods (Bayesian network, Mark’s decision-making process, etc.) are at the heart of many machines’ learning algorithms; these are ways of resolving uncertainty in real life.
Closely related are the statistical fields that provide different measurements (mean, median, variance, etc.), distributions (homogeneous, normal, binary, Poisson, etc.), and analytical methods (ANOVA, hypothesis testing, etc.) required to construct and test each model. Many machine learning algorithms are a continuation of statistical models.
Distributed computing
In most cases, a research project today involves working with large data sets. Since all processing is likely to take place on a Linux machine, you must have access to these tools.
Data modeling and evaluation
Data modeling is the process of estimating the basic structure of a particular data set to find useful models (correlations, clusters, managers, etc.) and to predict the characteristics of invisible cases (classification, regression, deviation analysis, etc.). A key element of this evaluation process is the continuous evaluation of the quality of a particular model.
You must select the appropriate accuracy or error measurement (loss before sorting, the sum of regression errors per square meter, etc.) and assessment strategy (training test partition, temporary checking of consecutive sequences, etc.). Repetitive algorithms learn to use errors that often occur directly (neural network redistribution) to refine models, so understanding these measurements is very important.
You should also be aware of the advantages and disadvantages of different methods and the many risks - that may be involved (bias and variability, over-estimation and under-estimation, missing data, data leakage, etc.). Statistics and machine learning challenges are a great way to get into different types of cases and their distinctions.
The future of machine learning
As a machine learning expert, you'll analyze data to tell a story and build knowledge for your team members. The analysis is done and presented by experts, for other team members, who can then make business decisions based on what is presented.
The audience of your production is human, though many of your daily tasks will include extensive work with advanced data sets and automation, your work is the endpoint of the software.
The faster you learn machine learning basics, the faster you can take your place in an exponentially-growing industry.
QuickStart’s AI & Machine Learning Bootcamp is designed to prepare students for next-level ML careers. You'll engage in labs, participate in hands-on projects, and work with legendary mentors who lead the world's top machine learning organizations. Take concrete steps toward a meaningful ML career in as few as 24 weeks, when you get started today.